- Solan Sync
- Posts
- [Latest AI Paper] MMLU-Pro: Advancing Language Model Benchmarks with Enhanced Reasoning Challenges
[Latest AI Paper] MMLU-Pro: Advancing Language Model Benchmarks with Enhanced Reasoning Challenges
Discover how MMLU-Pro elevates language model evaluations with complex reasoning tasks and robust question sets, pushing AI capabilities to new heights.
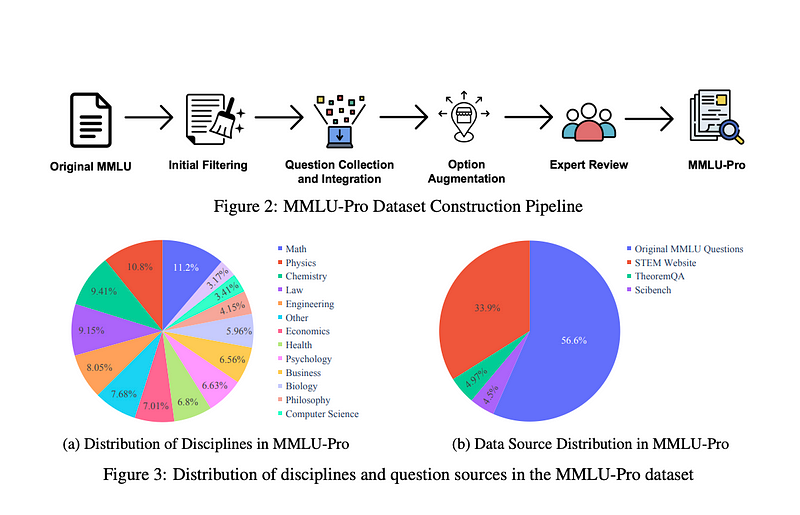
Recent advancements in large language models (LLMs) such as GPT-4, Gemini, and Claude have significantly pushed the boundaries of natural language processing. These models exhibit remarkable performance across a wide array of tasks, indicating substantial progress towards achieving expert-level intelligence, comparable to the top 10% of skilled adults. To accurately measure these advancements, comprehensive evaluation across diverse tasks is essential.
Currently, benchmarks like AGIEval, ARC, BBH, and MMLU are widely used to gauge LLM performance. Among these, the Massive Multitask Language Understanding (MMLU) benchmark has become a standard due to its extensive coverage and high quality. However, with LLMs nearing performance saturation on MMLU, a more challenging and discriminative dataset is necessary. This need has led to the creation of MMLU-Pro, an enhanced benchmark designed to elevate the assessment of language understanding and reasoning in LLMs.
Key Findings
MMLU-Pro builds upon the foundation of the MMLU benchmark but introduces several critical enhancements. The new benchmark focuses more on reasoning-intensive questions and expands the choice set from four to ten options, significantly increasing the difficulty level and minimizing the chances of correct guesses by luck. Additionally, MMLU-Pro eliminates trivial and noisy questions from the original MMLU dataset, ensuring a more robust evaluation.
Experimental results reveal that MMLU-Pro poses a considerable challenge for LLMs. Models experienced a substantial drop in accuracy, ranging from 16% to 33%, compared to their performance on MMLU. Moreover, MMLU-Pro demonstrated greater stability across different prompts, reducing sensitivity from 4–5% in MMLU to just 2%. This improvement highlights MMLU-Pro’s ability to provide a more consistent and reliable measure of a model’s true capabilities.
A notable finding is that models employing Chain of Thought (CoT) reasoning outperformed those using direct answering on MMLU-Pro. This contrasts with the original MMLU, where direct answering often yielded better results. This shift underscores the increased complexity and reasoning demands of MMLU-Pro questions.
Motivation and Objectives
The limitations of the MMLU benchmark stem from three main issues:
Limited Number of Distractor Options: The restricted number of distractor choices allows models to potentially exploit shortcuts without truly understanding the rationale.
Knowledge-Driven Questions: Predominantly knowledge-based questions require less reasoning, particularly in STEM subjects.
Presence of Unanswerable or Incorrectly Annotated Questions: These issues lower the benchmark’s effectiveness.
To address these limitations, MMLU-Pro introduces a more challenging and discriminative dataset, aiming to better track the progress of LLMs through complex reasoning questions and an increased number of distractor options.
Dataset Construction
MMLU-Pro spans 14 diverse domains, including mathematics, physics, chemistry, law, engineering, psychology, and health, encompassing over 12,000 questions. The dataset integrates questions from the original MMLU, high-quality STEM problems from online platforms, TheoremQA, and SciBench. Two rounds of expert reviews were conducted to reduce noise and ensure the accuracy and appropriateness of the questions.
The dataset construction involved initial filtering to remove overly simple questions and merging the previous 57 subject categories into 14 broader ones. This restructuring aimed to focus the evaluation on key areas of knowledge and reduce redundancy. Questions answered correctly by more than four models were considered too easy and excluded from the dataset.
Evaluation and Results
MMLU-Pro was used to evaluate more than 50 LLMs, including both open-source and closed-source models. The leading model, GPT-4o, achieved an accuracy of 72.6% on MMLU-Pro, indicating substantial room for improvement. In contrast, the top models on MMLU clustered around scores of 86–87%, highlighting the increased difficulty of MMLU-Pro.
MMLU-Pro’s discriminative nature is evident in the performance gaps between models. For instance, the gap between GPT-4o and GPT-4-Turbo widened from 1% on MMLU to 9% on MMLU-Pro, demonstrating MMLU-Pro’s ability to better distinguish between models with similar performances on MMLU.
Subject-Specific Insights
Performance disparities among models were more pronounced in computation and reasoning-intensive subjects like math and physics. Conversely, knowledge-intensive subjects like history and psychology exhibited a higher performance floor. Engineering and law consistently scored lower, indicating the need for further advancements in these areas.
Error Analysis
An error analysis of GPT-4o revealed that 39% of errors were due to flaws in the reasoning process, 35% stemmed from a lack of specific domain expertise, and 12% from computational errors. This highlights the challenges posed by MMLU-Pro and identifies areas for further research and model enhancement.
Conclusion
MMLU-Pro represents a significant advancement in benchmarking LLMs. By incorporating more challenging, reasoning-focused questions and increasing the number of distractor options, it provides a more robust and discriminative evaluation. MMLU-Pro not only raises the difficulty bar but also enhances the stability and reliability of model assessments, making it a valuable tool for tracking progress in the field of AI language capabilities.
The introduction of MMLU-Pro is a crucial step towards pushing the boundaries of what language models can achieve. As AI technology continues to evolve, MMLU-Pro will play a vital role in advancing our understanding and capabilities of LLMs.
Startup Ideas Inspired by Recent Advancements in LLM Benchmarks
1. AI Performance Analytics Platform
Advantages:
High demand for tools to evaluate AI model performance.
Opportunity to collaborate with leading AI research institutions.
Potential to set industry standards in AI benchmarking.
Disadvantages:
Requires deep expertise in AI and machine learning.
High initial development costs.
Competitive market with existing analytics solutions.
3-Month Action Plan for MVP:
Month 1:
Research and define key performance metrics and features for the platform.
Assemble a team of AI experts and software developers.
Develop a prototype of the platform focusing on core functionality.
Month 2:
Integrate basic benchmarking tools and test with existing LLMs.
Conduct initial user testing and gather feedback.
Refine and enhance the prototype based on feedback.
Month 3:
Develop a user-friendly interface and add advanced analytics features.
Begin marketing to potential early adopters (AI research labs, tech companies).
Plan and initiate a beta testing phase with select users.
Points to Explore for Validation:
Evaluate the current market demand and competition.
Identify key pain points in existing benchmarking tools.
Determine pricing models and potential revenue streams.
Understand regulatory and compliance requirements for handling AI data.
2. AI-Enhanced Educational Platform
Advantages:
Growing market for online education and AI-driven learning tools.
Potential to improve learning outcomes with personalized AI tutoring.
Can leverage the latest LLM advancements for interactive learning.
Disadvantages:
Significant content creation and curation required.
Need to continuously update the platform with latest AI models.
High competition from established educational platforms.
3-Month Action Plan for MVP:
Month 1:
Define the target audience and core subjects to cover.
Develop a basic platform architecture and select appropriate LLMs.
Create initial educational content and interactive lessons.
Month 2:
Integrate AI models for personalized learning paths and feedback.
Conduct pilot tests with a small group of users.
Collect and analyze feedback to improve the platform.
Month 3:
Enhance user interface and experience.
Develop marketing strategies and reach out to potential users.
Prepare for a wider launch with more subjects and features.
Points to Explore for Validation:
Assess the effectiveness of AI-driven learning compared to traditional methods.
Explore partnerships with educational institutions for content and credibility.
Identify potential challenges in scaling the platform.
Evaluate the long-term sustainability and updates required for the platform.
Unlock the Power of AI with Solan AI and SolanSync!
Are you ready to supercharge your AI learning journey? At Solan AI, we merge the power of GPT with Data Science to provide you with a revolutionary way to learn AI fast and effectively.
Explore our newsletter, where we’ve published six insightful articles to kickstart your AI education. Become a proficient AI applier with expert guidance and practical insights, all at your fingertips.
Join us as a paid subscriber for just $5 per month and gain access to the full LearnAI series and more exclusive content.
👉 Visit Solan AI to learn more. 👉 Subscribe to Solan Sync for the latest updates and insights.
Don’t miss out on the opportunity to elevate your AI skills with Solan AI and Solan Sync!
Thank you for reading this article so far, you can also access the FREE Top 100 AI Tools List and the AI-Powered Business Ideas Guides on my FREE newsletter.
The essential 100+ AI Tools For Creators & Entrepreneurs
Find awesome AI tools to make your work easierDive into the world of AI with these top-notch picks. These tools are for…solanai.gumroad.com
What Will You Get?
Access to AI-Powered Business Ideas.
Access our News Letters to get help along your journey.
Access to our Upcoming Premium Tools for free.
If you find this helpful, please consider buying me a cup of coffee.
Yuki is building an AI Prompt Generator Platform
Hey, I’m a Founder of @ai_solan | an AI Prompt Generator Platform | Web3 Enthusiast | Embracing Innovation and…www.buymeacoffee.com
✅ Stop paying subscription. Try Awesome AI Tools & Prompts with the Best Deals
🧰 Find the Best AI Content Creation jobs
⭐️ ChatGPT materials
💡 Bonus
🪄 Notion AI — If you are fan of Notion and solo-entrepreneur, Check this out.
If you’re a fan of notion this new Notion AI feature Q&A will be a total GameChanger for you.
After using notion for 3 years it has practically become my second brain it’s my favorite productivity app.
And I use it for managing almost all aspects of my day but my problem now with having so much stored on ocean is quickly referring back to things.
Let me show you how easy it is to use so you can ask it things like
“What is the status of my partnership” or “How many books have I read this year?” and this is unlike other AI tools because the model truly comprehends your notion workspace.
So if you want to boost your productivity this new year go check out Notion AI and some of the awesome new features Q&A!
Reply