- Solan Sync
- Posts
- [What’s RAG?] Bridging Knowledge Gaps: The Role of Retrieval-Augmented Generation(RAG) in Enhancing AI
[What’s RAG?] Bridging Knowledge Gaps: The Role of Retrieval-Augmented Generation(RAG) in Enhancing AI
Discover how Retrieval-Augmented Generation (RAG) is transforming AI by integrating real-time data for more accurate and relevant responses. Learn about its impact across industries.
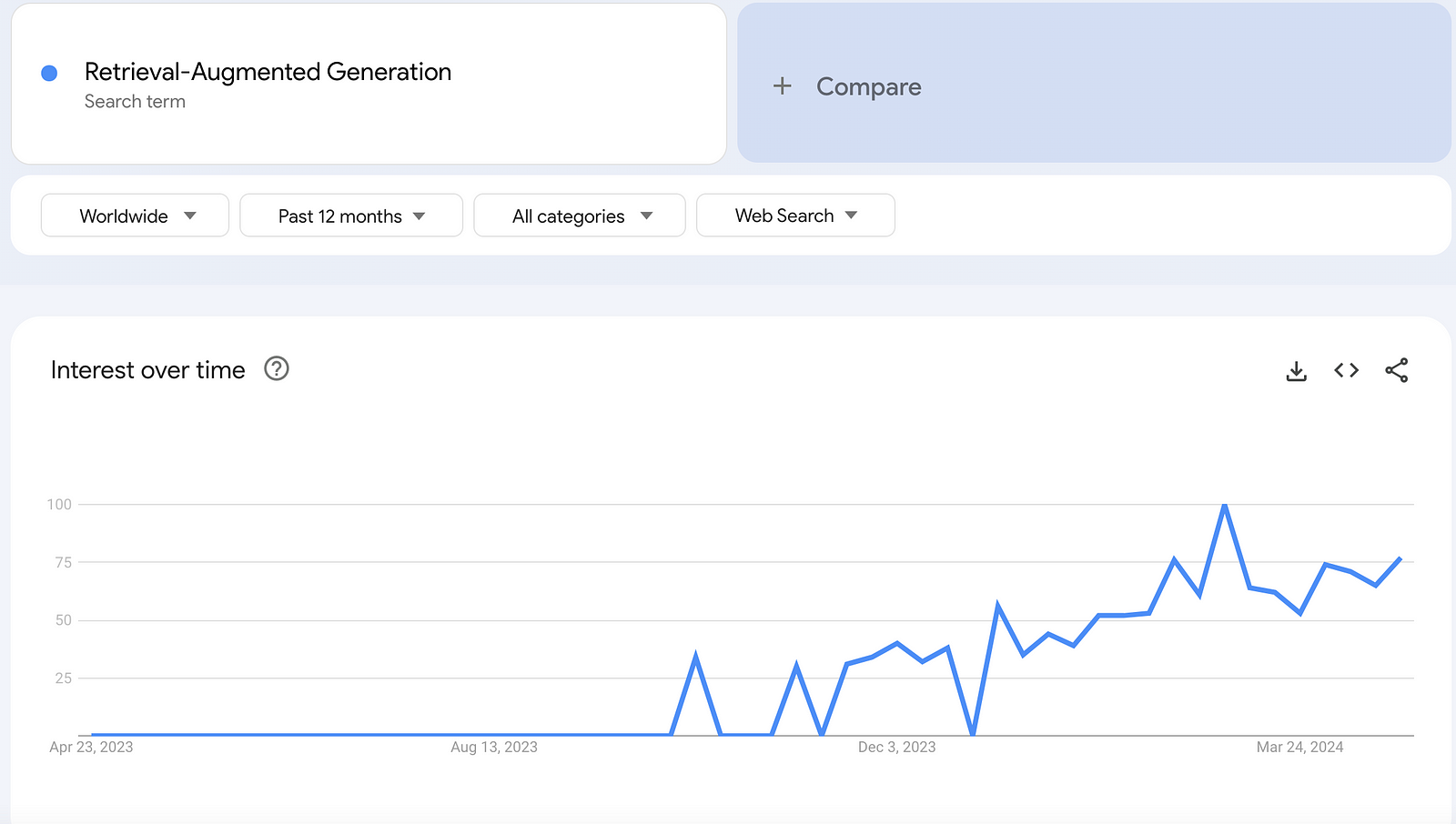
That’s right.
With an explosive entry of OpenAI and their revolutionary product, ChatGPT, the world of AI comes crashing into your life.
Still, though, many essential terms and practices go unseen by the new or casual user.
Retrieval-Augmented Generation(RAG) is a technique that enables large language models, such as ChatGPT, to access more relevant retrievable context along with the small portion that can be offered to them for training.
At its heart, RAG allows systems that would be useful in only a few narrow domains to be much more generally capable across many domains.
In this article, we’ll explore:
What is the RAG?
Why is RAG important
How RAG Works
Examples of use for RAG
How to test the RAG approaches: reasons why this should be explored
Let’s dive in!
What is RAG?
Simply, RAG is essentially an integrated LLM (Large Language Models) like GPT-4 with external databases or APIs, thus allowing real-time information retrieval to give updated and more informed responses.
Another easy way to look at this is to think of RAG as a combination of a librarian and a skilled writer.
This means that an API or database has very detailed knowledge to draw from, while ChatGPT is able to explain a huge number of topics it was trained to write about.
Basically, put together, the skilled writer (ChatGPT) can now explain all the additional information given to it by the librarian (a database/API).
Generally put, RAG increases the knowledge base of an LLM exponentially and helps to ground an LLM with verifiable facts. The “retrieval” part of RAG can actually scrape websites and documents for “context” relevant to the user prompt.
The retrieved context is given to the LLM (ChatGPT) depending on the retrieval sophistication of the method/system. The LLM (ChatGPT) therefore answers the user prompt by using the pre-existing knowledge base and the retrieved context.
The retrieve function can be allowed access to any website or browsing, database, individual document, and other external source of information.
Take one last look at an example of analogy for this basis of RAG before we move on. If the paper or research is asking the writer to find books on the subject, she can usually only give the writer one or two books, not more. It tests the skill of the librarian in that he has to determine what books would provide the answers which the writer needs.
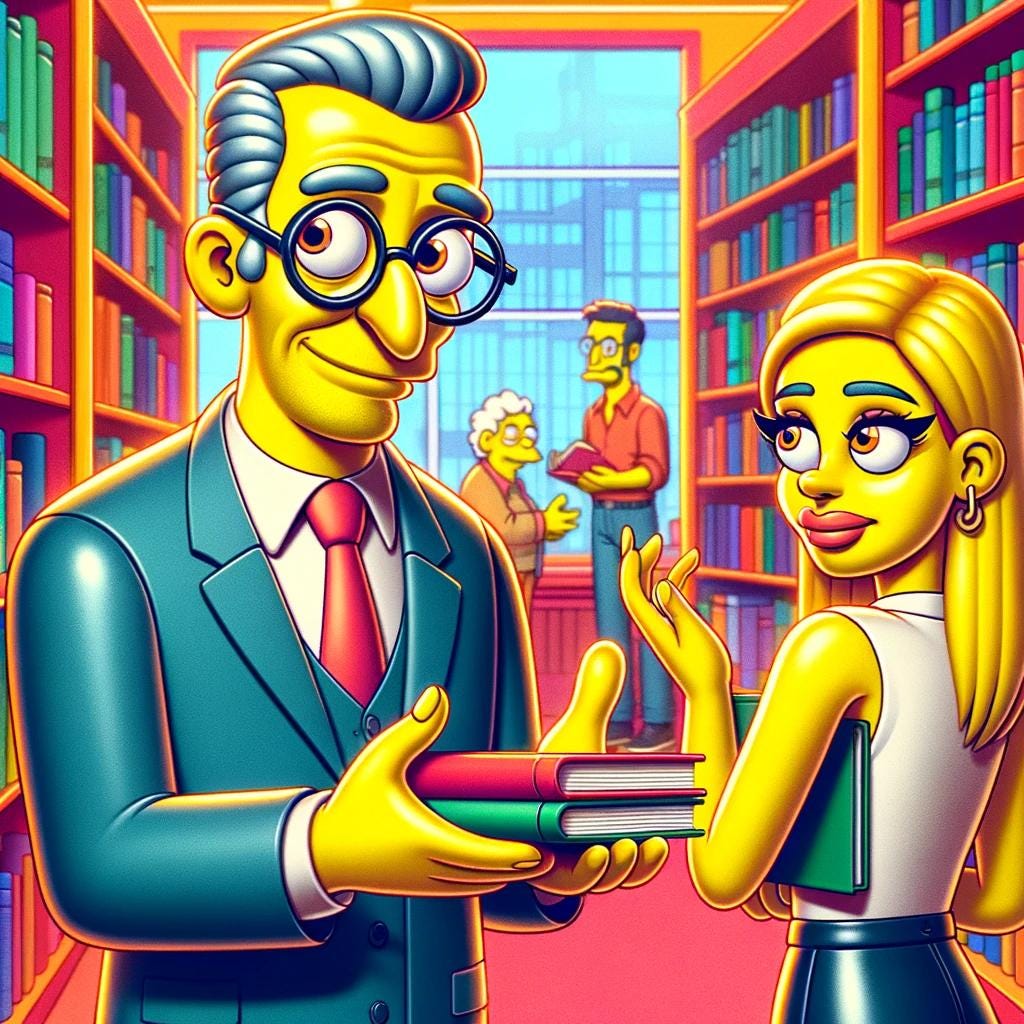
Why is RAG important?
Generation through retrieval-Augmented is a powerful tool in itself, unlocking the complete potential of LLMs. All existing leading models of LLMs are trained over huge, yet outdated information. OpenAI GPT-4, for example, cuts off training data from the year 2021 and hence makes it, for two years, RAG models are necessary to fill in the knowledge and data gaps.
RAG also helps correct or precise responses. When generating a response to a user query, the model would use the retrieval element in order to seek out responses, which had been retrieved for its generation of a response. Origin of information could also be shared with the user so that the user may search for possible bias or inaccuracies while an answer is being provided.
I am referring to the “context limits” for LLMs earlier in this post. In short, “context” refers to the quantity of text or information that the model is allowed to use to come up with a response. This quantity of context is token-based. Tokens don’t have a fixed value, and they can cover anything from a single character up to whole words. (Yes, weird — learn more about the tokens in OpenAI’s visual tokenizer.)
How RAG Works
Data Retrieval: When a query is posed, RAG begins by fetching pertinent information from a vast dataset. This is similar to a search engine looking up relevant articles or data points.
Response Synthesis: The retrieved data is then seamlessly incorporated into the model’s response generation process. This allows the LLM to craft responses that are not only contextually relevant but also enriched with up-to-date and specific information.
Output Integration: Finally, the model combines its pre-trained knowledge with the newly retrieved information to produce a comprehensive and informed response.
Use Cases of RAG in LLMs
Healthcare: In medical diagnostics, RAG can provide LLMs with the latest research data or clinical findings, aiding healthcare professionals in making informed decisions.
Legal Assistance: For legal professionals, RAG can retrieve relevant case laws or statutes, helping in the preparation of cases or legal opinions.
Customer Support: In customer service, RAG can pull information specific to a customer’s query, providing personalized support without manual lookup.
Education and Research: Students and researchers can benefit from RAG-powered LLMs which can provide citations, current data, and summaries from a wide range of academic papers and sources.
Financial Services: In finance, RAG can enhance decision-making by providing real-time market data, financial reports, and economic indicators integrated within the model’s responses.
Challenges and Considerations
Data Reliability: The effectiveness of RAG heavily depends on the quality of the data retrieved. Ensuring data accuracy and timeliness is crucial.
Privacy and Security: Handling sensitive information through external data sources requires robust security measures to protect data integrity and privacy.
Complexity and Cost: Implementing RAG involves additional computational resources and complex infrastructure, which might increase operational costs.
RAG transforms traditional LLMs from static knowledge repositories to dynamic, intelligent systems capable of accessing and integrating a vast range of up-to-date information.
As AI continues to evolve, the integration of retrieval mechanisms like RAG will be pivotal in realizing the full potential of AI applications across various domains.
Here’s how you can structure your document or presentation to explore the timely relevance of Retrieval-Augmented Generation (RAG), identify the opportunities it presents, and introduce several business ideas leveraging this technology.
Why Now
Discuss the current landscape and developments in AI that make this the right time to invest in RAG technology. Highlight the growing need for real-time, accurate information processing across industries and how recent advancements in machine learning and data retrieval technologies have made RAG more feasible and effective than ever before.
The Opportunity
Detail the specific opportunities that RAG presents for businesses and organizations. Focus on its ability to enhance decision-making, improve customer interactions, and create more dynamic and responsive AI systems. Emphasize the competitive advantage that RAG can provide in a data-driven market where timely and relevant information is a critical asset.
Business Idea #1: RAG-Enhanced Customer Support Platform
Description: Develop a platform that uses RAG to provide real-time customer support, leveraging up-to-date information retrieval to answer queries more effectively and personalize interactions.
Advantages: Improves customer satisfaction with accurate, relevant responses; reduces response times; decreases workload on human agents.
Challenges: Requires integration with various data sources; maintaining data privacy and security.
Business Idea #2: RAG-Powered Legal Research Tool
Description: Create a tool that uses RAG to assist legal professionals by quickly retrieving case laws, precedents, and statutes relevant to ongoing cases or queries.
Advantages: Saves time in legal research; increases the accuracy of legal advice; can be tailored to different jurisdictions.
Challenges: Needs access to comprehensive and continually updated legal databases; complex categorization of legal texts.
Business Idea #3: Dynamic Content Creation for Education
Description: Utilize RAG to develop educational tools that provide teachers and students with the latest information, scholarly articles, and data for a more enriched learning experience.
Advantages: Keeps educational content up-to-date; enhances learning with access to the latest research and data; personalized learning experiences.
Challenges: Integrating diverse educational resources; curating content for different educational levels and subjects.
These headings and descriptions should provide a clear, organized way to present the importance of RAG, its potential impact, and specific innovative business ideas that exploit this cutting-edge technology.
Thank you for reading this article so far, you can also access the FREE Top 100 AI Tools List and the AI-Powered Business Ideas Guides on my FREE newsletter.
What Will You Get?
Access to AI-Powered Business Ideas.
Access our News Letters to get help along your journey.
Access to our Upcoming Premium Tools for free.
If you find this helpful, please consider buying me a cup of coffee.
📚 Try Awesome AI Tools & Prompts with the Best Deals
🧰 Find the Best AI Content Creation jobs
⭐️ ChatGPT materials
💡 Bonus
🪄 Notion AI — If you are fan of Notion and solo-entrepreneur, Check this out.
If you’re a fan of notion this new Notion AI feature Q&A will be a total GameChanger for you.
After using notion for 3 years it has practically become my second brain it’s my favorite productivity app.
And I use it for managing almost all aspects of my day but my problem now with having so much stored on ocean is quickly referring back to things.
Let me show you how easy it is to use so you can ask it things like
“What is the status of my partnership” or “How many books have I read this year?” and this is unlike other AI tools because the model truly comprehends your notion workspace.
So if you want to boost your productivity this new year go check out Notion AI and some of the awesome new features Q&A!
Reply